Wie fördert man lernen?
Die folgenden acht Eigenschaften mögen einen nicht überraschen, jedoch möchte ich mich damit beschäftigen, warum diese so wichtig sind und wie wir sie für die Gestaltung von Lehranwendungen anwenden können.
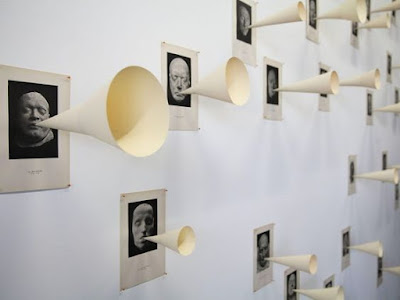
1. Mulitmodales Lernen
Um neues Wissen gut abrufbar abzuspeichern, empfiehlt es sich, verschiedene Modis anzusprechen. Beispielsweise einen gesprochenen Vortrag durch bildhafte Abbildungen zu unterstützen. Hierbei werden mehrere Assoziationsketten und Verknüpfungen zu einer Information gelegt. Da das Ansprechen von mehreren Sinnesorganen nach [1] auch eher in Erinnerung bleibt, ist es sinnvoll für die Gestaltung von Für interaktive Anwendungen
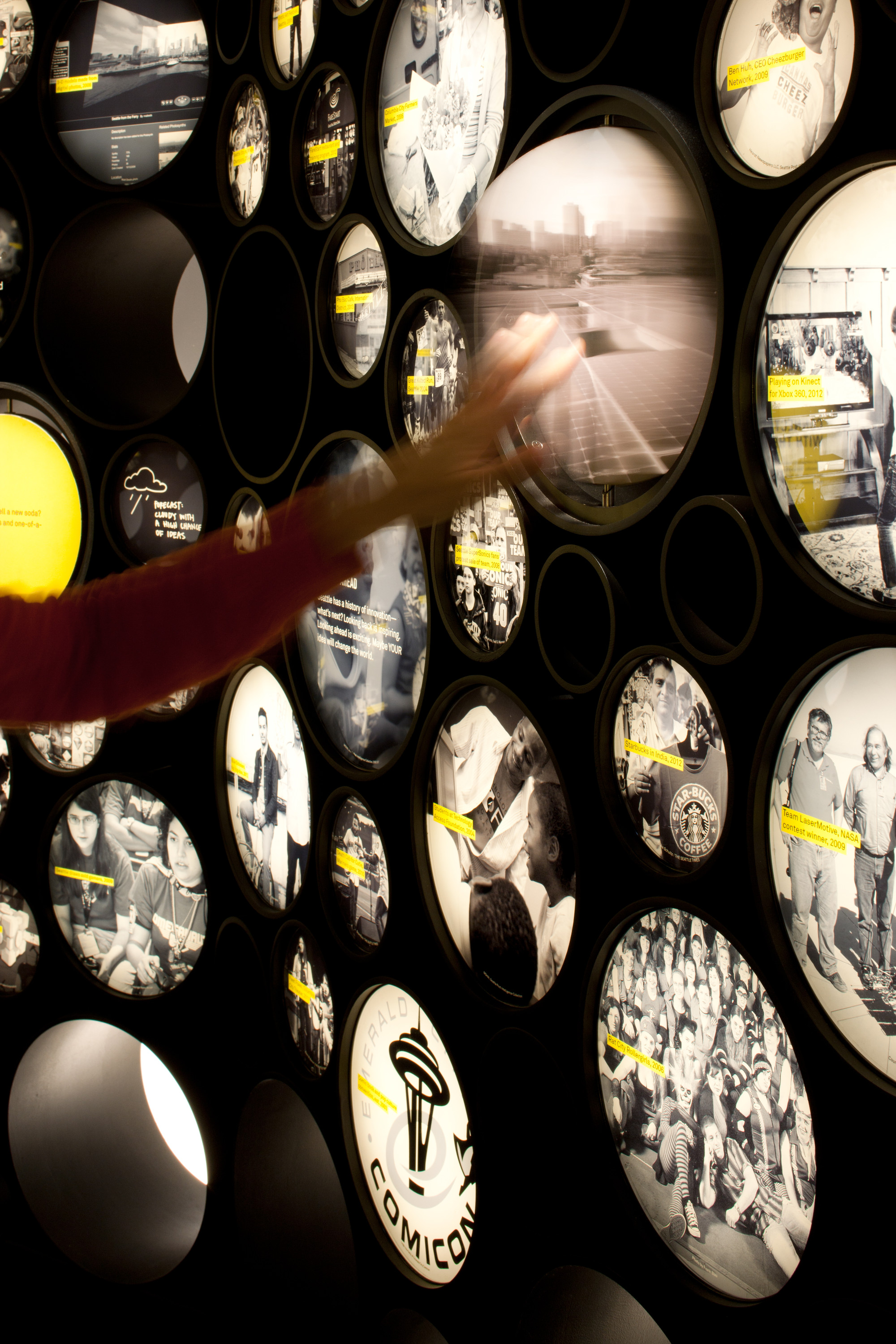
2. Semantische Einbettung
Da das Gehirn als semantisches Netzwerk aufgebaut ist, ist es hilfreich, wenn verschiedene Queues auf Wissen zugreifen, bzw. wenn mehrere Verknüpfungen zu einer Information führen. Bereits vorhandenes Wissen, dient hierbei als Gedankenstütze für neu erlerntes. In diesem Netzwerk können neue Inhalte an zuvor erlerntem angeknüpft werden. Es macht somit Sinn, neue Themen mit wohlmöglich bekanntem Wissen einzuleiten.

3. Emotionen
Emotionen haben eine starke Auswirkung auf unsere kognitiven Fähigkeiten, sowie Wahrnehmung, Aufmerksamkeit, Lernen, Gedächtnis und das Lösen von Problemen. Sowohl positive wie auch negative Emotionen können Lernförderlich sein. Beispielsweise wirken sich Belohnungen sowie auch milder Druck und Bestrafungen auf den Lernerfolg aus.
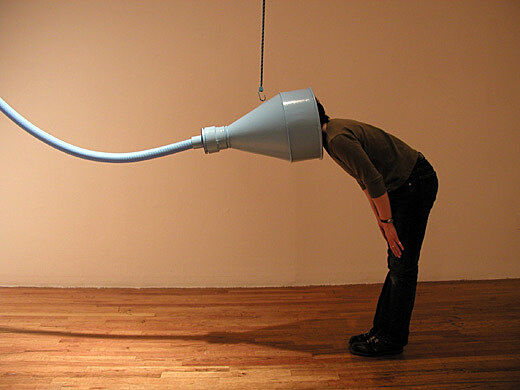
4. Tiefe der Verarbeitung
Je tiefer etwas gelernt wird, also wie häufig, wie elaboriert, wie detailliert, desto eher ist die neu gewonnene Information effizient abrufbar. Wenn Verbindungen zu anderen verwandten Themen hergestellt werden und das neu erlernte im Kontext gesehen wird, wird der Effekt verstärkt, da es zu einer tieferen Auseinandersetzung kommt.

5. Relevanz
Das angestrebte Wissen sollte eine gewisse persönliche Relevanz haben, wie beispielsweise das Interessenfeld des Nutzers oder eine allgemeine Relevanz beinhalten.
Quellen
[1] Max-Planck Gesellschaft: Lernen mit allen Sinnen, 05.02.2015
https://www.mpg.de/8930937/vokabel-lernen-gesten
Abb.1 | https://blog.orselli.net/2020/11/rewind-are-exhibit-timelines-so-boring.html
Abb.2 | https://images.adsttc.com/media/images/52cd/fd12/e8e4/4e34/3700/002b/large_jpg/11053_00_MOHAI_Center_For_Innovation_N18.jpg?1389231329
Abb. 3 | http://everyoneishappy.com/
Abb. 4 | https://whitney.org/events/sound-accumulation
Abb. 5 | https://i.pinimg.com/564x/39/19/b3/3919b3b7470caad88293583b4f7c3940.jpg