For the research on the field of artificial intelligence I collected lots of information from different websites and videos. In the beginning I had problems to gather everything in a nice manner. Positioning images in a Microsoft word document seems like an undoable task. Pen and paper didn’t allow me to copy and paste anything. With Indesign I started layouting stuff which didn’t need a layout. After trying out some tools and being frustrated with the restrictions, I was searching for a tool which could help me to save links and videos, structure information, add text, thoughts and images. It should be easy to use in the process and the output should look appealing without too much design effort. After trying out some online note-tools, I found Milanote [1]. You can have a look at my collection to see more examples for AI driven designs and articles [11]. Milanote is a free online tool for organizing creative projects. It shall help gathering information and structuring it however its desired. I fell in love instantly.
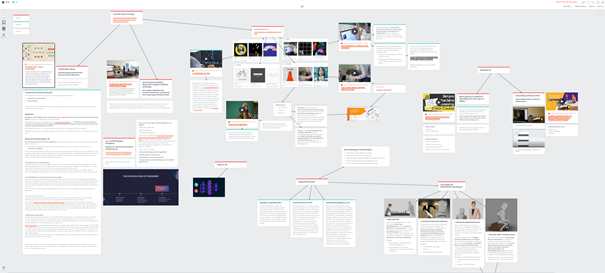
There is a lot to know about the typology, methods and kinds of AI. In the last blogpost I already explained the difference between machine learning, artificial intelligence and neural networks. The AI systems we know today are based on machine learning. How a simple machine learning algorithm works is not too difficult to understand. There are tons of YouTube videos which explain the basics, I recommend the video of 3Blue1Brown [2] because of it’s visual explanation. But anything will do.
I took a closer look at how I could use artificial intelligence in my own field of interest. As an interaction designer, there are many intersections where AI can help to create. I came across the website of Google AI experiments [3] where different AI projects are shared. “AI Experiments is a showcase for simple experiments that make it easier for anyone to start exploring machine learning, through pictures, drawings, language, music, and more.” It says on the website. It’s collection of work from Google teams and other creative teams in different fields which used AI to find a solution to problem. You find AI examples for learning, drawing, writing, music and other experiments. Just the shear sum of creative work built with AI struck me.
I was especially impressed by the “teachable machine” by the Google Creative Lab [6]. They invented a free online tool, where you can build your own machine learning model in such an easy way… to be honest- it feels somehow rude how easy this tool makes machine learning seem. The video was very inspiring, showing all kinds of solutions and ideas built with pattern recognition. I think this is a huge step in the development of AI and machine learning. I tried the tool if it can spot if I’m wearing glasses or not. First you need to gather pictures of what the method shall recognize. Taking a few selfies of myself wasn’t too difficult. Secondly, by just clicking a button (yes just clicking a button!!) you can train your model and boom that’s all.
This opens up a whole new world to non-programmers and will allow thousands of creative people stepping into the field of machine learning/AI. I have the feeling that using this online tool for a own project might still be more difficult that it seems in the first place, since you need to set up the communication between your model and your code, but still- I’m impressed. Furthermore, this new technique of collecting data of an object opens up a whole new perspective. One of the annoying parts of training a machine learning code was, that you had to feed the model with tagged data. We all know those reCAPTCHA pictures from Google, where you need to click on the pictures which show traffic lights, cars, busses, road signs and stuff like that. What we are doing there is not only a proof for a login, but we are actually feeding and AI with very precious information [4]. (I sometimes click on the wrong images on purpose to confuse Google)
Furthermore, I made a list of how AI could be used in our field of work. This collection is driven a lot by which technologies we used in our first semester.
- Use pattern recognition and build physical systems with Arduino
– Use an Arduino to build a hardware solution where physical things are triggered.
– Get the input of an event via image (computer vision) or via sound.
– React to that event with your Arduino device.
– Example: showed in the video of the teachable machine [5]. Could be used to switch on a light of a disabled, open the little door when the system sees the dog approaching, sort trash by filming the trash etc. - Use pattern recognition to control an object in virtual space
– Use Unity to control an object in a virtual space.
– Can track hand gestures or body movement to navigate or manipulate within a virtual 2D or 3D space.
– Can use for interactive applications in exhibitions
2.1 The more neural activity your brain has, the more likely you will remember something. If you get the body to move, you can trigger the muscle memory and the user might find it easier to remember your content. For example: teach music theory not only with information or sound but using the gap between your fingers to visualize harmony theory.
2.2 A higher immersion can lead to more empathy. For example if you made the experience of being an animal in a burning jungle with virtual reality for instance, you might feel more empathy for this concern. A lived experience is more likely to influence emotionally rather than just telling a person that animals are dying in fire. - Draw images with sound
– Create “random” images which are drawn by incidents in the real world.
– For the implementation you could use processing or p5.
– Example: you could record with your Webcam, film the street and trigger an event when a blue car is driving by. This could change how a picture is drawn by code. You could also use certain sounds instead. - Visualizing data
– Collect data and visualize it in images or in a virtual space.
– Huge amounts of data can be classified and structured by machine learning to create strong Infographics.
– Data can be very powerful and reveal insights which you wouldn’t think of. There are good examples where well-structured meta data showed coherences, which didn’t seem related to the data itself. An episode of the design podcast 99% invisible talked about how a list of e-mails within a company showed information of who is a manager and who was probably dealing with illegal and secret projects – without reading one e-mail [7]. Moreover, David Kriesel gives with his presentations an impression of how powerful meta data is [8]. With the power of machine learning and AI we could reveal information which don’t seem obvious in the first place.
– Example:
https://experiments.withgoogle.com/visualizing-high-dimensional-space - UI design, recommendations and personalization
– Use machine learning (ML) in your UI to make navigation easier and quicker.
– Personalize systems for your user and create experiences where the user can move freely within your application
– Best practice found in article [9]:
5.1. Count decisions as navigation steps
Count how many decisions need to be made for navigating though system. Reduce them with ML. The ML-generated suggestions shouldn’t make the user evaluate the options, otherwise the ML doesn’t make any sense here.
5.2. A predictable UI is necessary when the stakes are high
Do not use ML for critic navigation/finding. Human works best in such cases. Consider using it for browsing and exploration.
5.3. Be predictably unpredictable
Basically, hide the new ML feature. Think it depends on use case.
5.4. Make failure your baseline
ML will make mistakes. Built the system that if mistakes happen, it doesnt take longer to erase them rather than just doing the job on your own in the first place. - Use AI for creative exchange
– Use AI as a communication in creating new concepts.
– AI is good in making links and connections to similar fields. Also, it’s good at bringing randomness into the game.
– Example of writers which chat with AI to boost their ideas. Since ai is built with a neural network its kind of works like our brain, so it’s capable of bringing fascinating ideas for the field it’s programmed for. And since it’s a machine and not a human it can bring new perspectives into thinking (see youtube “Prescursors to a Digital Muse” below).
– Example: The AI for the game GO, played a move which seemed like a bad one to a human but maximized the winning probability since it was interested in winning the whole game and not conquering as many fields as possible. Professional GO players examined the new thinking of the game which is played since the 4th century, with a new perspective [10]. - Get rid of repetitive tasks
I was so fascinated when I saw how the new iPhone does all the photo editing which I used to do in hours of work, automatically. Of course, it does mistakes and is not as accurate, but come on, who enjoys cropping of a curly haired person in Photoshop. Using a cropped image and putting it somewhere else is the fun part, not the cutting out. At least for me. When such tasks are done by a machine, we can concentrate on all the other ideas we have with that curly-haired-person-image.
On the search on where AI is and where designers are, you could often read about the fear that AI will take away the jobs of designers. Since AI is capable of doing a lot of work which was dedicated to designers for a long time, it’s definitely true in some ways. But we need to evolve and adapt to technology. A lot of frustrating and repetitive tasks can be done by the machine, take advantage of this and start creating from that point. We can create much larger scaled projects when we can deal with such technologies.
- Free tool for collecting notes and structuring ideas:
https://milanote.com/ - Machine Learning tutorial:
https://www.youtube.com/watch?v=aircAruvnKk&t=3s&ab_channel=3Blue1Brown - Google AI experiments:
https://experiments.withgoogle.com/collection/ai - Article on how recaptcha images help Googles algorithms:
https://medium.com/@thenextcorner/you-are-helping-google-ai-image-recognition-b24d89372b7e - The teachable machine promotion video:
https://www.youtube.com/watch?v=T2qQGqZxkD0&feature=emb_logo&ab_channel=Google - The teachable machine website:
https://teachablemachine.withgoogle.com/ - Interesting podcast – the value of data:
https://99percentinvisible.org/episode/youve-got-enron-mail/ - Interesting presentation on the power of data mining:
https://www.youtube.com/watch?v=-YpwsdRKt8Q&t=2800s&ab_channel=media.ccc.de - AI in UI design
https://design.google/library/predictably-smart/ - Documentary of the Alpha Go AI
https://www.youtube.com/watch?v=WXuK6gekU1Y&ab_channel=DeepMind - Collection of articles, example videos and background information
https://app.milanote.com/1KX8J41TAgBr12?p=d7PvzxFcpuX